Articles
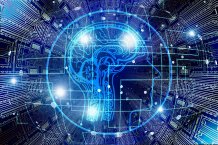
Machine learning algorithms can handle a far greater range of predictive factors
‘Algorithms 22% more accurate at predicting welfare dependency’
Artificial intelligence is a fifth more accurate at predicting whether individuals are likely to become long-term recipients of benefits.
A new method of predicting welfare dependency, developed by Dr Dario Sansone from the University of Exeter Business School and Dr Anna Zhu from RMIT University, could save governments billions in welfare costs as well as help them make earlier interventions to prevent long-term economic disadvantage and social exclusion.
Their study found that machine learning algorithms, which improve through several iterations and use of big data, are 22% more accurate at predicting the proportion of time individuals are on income support than the standard early warning systems.
The researchers were able to apply the off-the-shelf algorithms to the entire population of people enrolled in the Australian social security system between 2014 and 2018.
This included demographic and socio-economic data of anyone who received a welfare payment from Australia’s social security system Centrelink, whether on the grounds of unemployment, disability, having children, or being a carer, a student or of pensionable age.
The algorithm used a 1% sample of the roughly five million people registered in the system aged 15-66 in 2014 and followed that sample for the next three years, monitoring whether those individuals were still receiving income support payments.
It then compared the results to current methods of predicting welfare dependency based on profiling indicators such as sex, age and education, income support history, migration status, marital status, and state of residence.
They found that machine-learning algorithms were 22% more effective at predicting future income support than the best performing profiling benchmark methods.
According to the authors’ calculations, those individuals who were predicted to be long-term welfare claimants using machine learning algorithms cost the Australian State an additional $1bn AUS in welfare payments, the equivalent of around 10% of the amount it spends annually on unemployment benefits.
This greater accuracy is attributed to how machine learning algorithms can handle a far greater range of predictive factors (around 1,800 in total), which reflects the complex processes underlying welfare dependency.
The researchers say their approach is aimed at complementing existing early intervention programmes targeting long-term welfare receipt.
To implement these programmes, governments need to know which individuals are most at-risk – a role that the authors say can be ably fulfilled by machine learning algorithms.
The researchers add that these improved predictions may reduce conscious and unconscious biases common in human decision-making.
And crucially, the approach would be relatively low-cost to implement since it makes use of data already available to caseworkers.
Dr Dario Sansone, a Lecturer in Economics at the University of Exeter Business School, said: “Governments are increasingly using machine learning to tackle social problems and to make resource-allocation decisions. For example, it has been used to help judges to improve bail-granting decisions, schools to identify students at risk of dropping out, and surgeons to screen patients for hip-replacement surgery.
“We found that the size and richness of the dataset on social security enrolees makes it ideal for a machine learning application, allowing the algorithms to achieve high performance by detecting subtle patterns in the data and by identifying new powerful predictors.
“However, we do not believe algorithms should replace human expertise but rather act as its complement. Caseworkers could focus their attention and time providing a personalised service and targeting the appropriate support to individuals that the algorithm identifies as most at risk.”
Using Machine Learning to Create an Early Warning System for Welfare Recipients, by Dr Dario Sansone and Dr Anna Zhu, is published as a working paper in the IZA Institute of Labor Economics discussion paper series.
Date: 22 June 2021