Articles
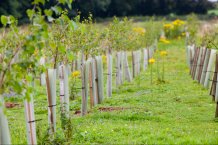
AI-enhanced decision support
systems for treescapes in the UK
IDSAI Funding for Pilot Projects Announced
The IDSAI is delighted to announce that five new research projects have been selected to receive an IDSAI Research Award or Exeter-Turing Pilot Research grant from the latest round of applications. These five awards join a previous project: detecting ancient hillforts, that was delayed due to Covid. All six projects will start in January 2021.
IDSAI Research Awards and Turing-Exeter Pilot Research Grants aim to support early-phase development of ideas for innovative, interdisciplinary data science and AI research across Exeter. Academic members of staff from all Colleges were invited to apply for the time of an IDSAI Research Fellow to work with them on a pilot research project. Congratulations to all those involved in the projects from five Colleges who were successful in a very competitive selection process:
1. Multimodel Techniques for the Study of Sexism in Political Institutions
Description: Sarah Child’s “Good Parliament” Report of 2016, which focused on making the UK House of Commons more inclusive especially for women, first recommendation was that “unprofessional, sexist and exclusionary language and behaviour should have no place in the House”. This sexist behaviour creates a barrier to the full participation of women in policymaking and decreases democratic quality. Therefore, it is crucial to study when, where and how sexism occurs in political institutions. Given that sexism is not one behavioural trait but can be exhibited in many ways (including through voice and gestures), the aim of this project is to explore the feasibility of developing a multimodal technique (relying on text, image and audio) to measure sexist language and behaviour in the House of Commons.
2. A machine-learning approach to classifying neuron type
IDSAI Research Fellow: DrRavi Pandit
3. AI-enhanced decision support systems for treescapes in the UK
IDSAI Research Fellow: DrBertrand Nortier
For each element of (i) and (ii) different research communities have models for their piece of the process. All models are interconnected and can be computationally intensive. To provide policy support, the project aims to run this network of models, propagating their uncertainties over a continuum of policies, in order to present decision makers (DM) with a subspace of policies that are consistent with their stated targets for CO2 sequestration and illustrate trade-offs with costs and other ecosystem services. DM may have an ill-formed prior understanding regarding the trade-offs they might accept across impacts, so rather than using a multi-objective optimisation, the vision is to deliver a mapping of the whole policy space for DMs to explore in real time, offering the potential for radical improvements in policy.
4. Identifying drug-vaccine interactions in electronic health record data using machine learning
IDSAI Research Fellow: DrOliver Stoner
Some of the most common prescription drugs in the UK are medications to treat high blood pressure, statins to treat or delay cardiovascular disease, proton pump inhibitors for heart-burn and acid related disorders, metformin to delay or treat diabetes and non-steroidal anti-inflammatory drugs. A number of these medications are also known to have modulating effects on the immune system that could either inhibit or enhance the immune response when patients receive routine vaccinations. The project team aims to use machine learning approaches to facilitate a data-driven exploration of drug-vaccine interactions to identify candidates for future investigation. This data-intensive screening approach has the potential for rapid deployment at scale to validate interactions with known mechanisms as well as contributing to the discovery of new targets. This proposed project combines methodology development with delivering clinically-relevant results for influenza vaccination.
5. Transfer learning for clinical applications
IDSAI Research Fellow: DrBertrand Nortier
6. Automated Detection of Hillforts in South West England Using a Machine Learning Approach
IDSAI Research Fellow: Dr Dmitry Kangin
Despite the recognition of their great potential the development of automatic detection methods applied to archaeological remote sensing is still in its infancy and limited to very simple morphologies. However, the increasing availability of remote sensing data of higher resolution, higher acquisition frequency and lower cost, demands a paradigm shift, where traditional man-made identification and mapping of archaeological sites cannot be the only option available.
The project will test the extent to which existing algorithms developed for other purposes can assist the detection in airborne LiDAR datasets of hillforts. The project proposes a meaningful solution to protect archaeological heritage and fight the rate of its destruction due primarily to development and climate change globally. If successful, this approach could be expanded and applied to other case studies involving similar archaeological objects elsewhere; other types of archaeological features; potentially other types of datasets (e.g. multispectral aerial and satellite imagery). This will be highly relevant in particular for cultural heritage management by national and local agencies in Britain and beyond.
The next round of IDSAI Research Awards is due to open in spring 2021. Please look out for more information about the Awards here.
Date: 20 January 2021
Date: 4 May 2020